Some of you are probably going to get into an automobile today.
Are you insane?
The National Highway Traffic Safety Administration has released its latest projections for traffic fatalities in 2022, estimating that 42,795 people died in motor vehicle traffic crashes.
From https://www.nhtsa.gov/press-releases/traffic-crash-death-estimates-2022
When you have tens of thousands of people dying, then the only conscionable response is to ban automobiles altogether. Any other action or inaction is completely irresponsible.
After all, you can ask the experts who want us to ban biometrics because it can be spoofed and is racist, so therefore we shouldn’t use biometrics at all.
I disagree with the calls to ban biometrics, and I’ll go through three “biometrics are bad” examples and say why banning biometrics is NOT justified.
- Even some identity professionals may not know about the old “gummy fingers” story from 20+ years ago.
- And yes, I know that I’ve talked about Gender Shades ad nauseum, but it bears repeating again.
- And voice deepfakes are always a good topic to discuss in our AI-obsessed world.
Example 1: Gummy fingers
My recent post “Why Apple Vision Pro Is a Technological Biometric Advance, but Not a Revolutionary Biometric Event” included the following sentence:
But the iris security was breached by a “dummy eye” just a month later, in the same way that gummy fingers and face masks have defeated other biometric technologies.
From https://bredemarket.com/2023/06/12/vision-pro-not-revolutionary-biometrics-event/
A biometrics industry colleague noticed the rhyming words “dummy” and “gummy” and wondered if the latter was a typo. It turns out it wasn’t.
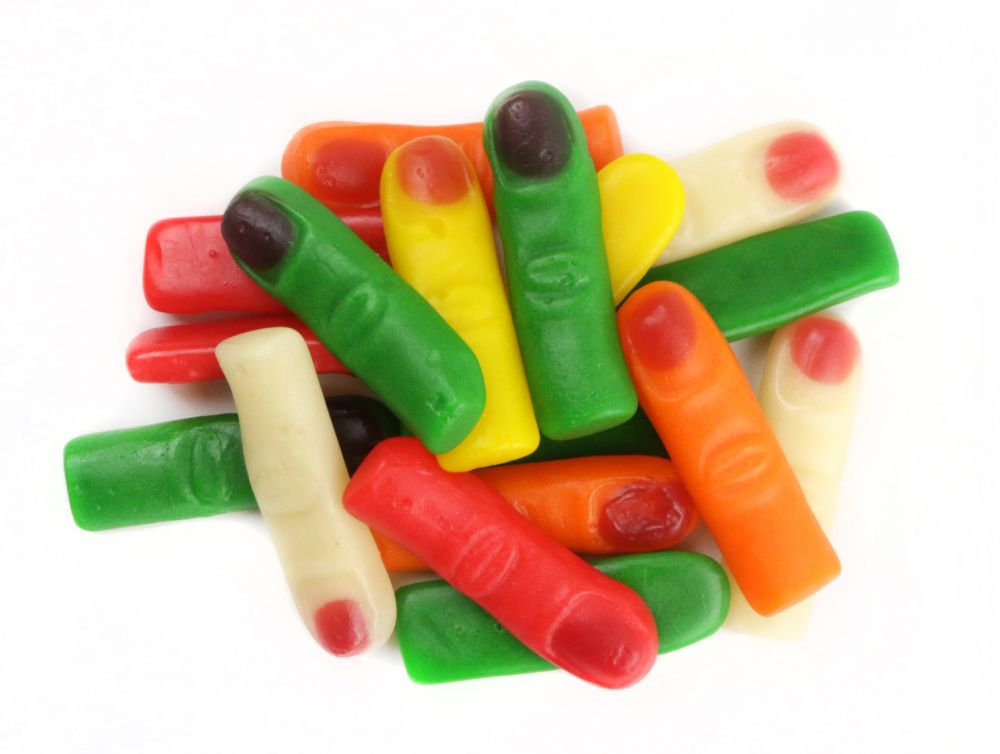
Back in 2002, researcher Tsutomu Matsumoto used “gummy bears” gelatin to create a fake finger that fooled a fingerprint reader.
Back in 2002, this news WAS really “scary,” since it suggested that you could access a fingerprint reader-protected site with something that wasn’t a finger. Gelatin. A piece of metal. A photograph.
Except that the fingerprint reader world didn’t stand still after 2002, and the industry developed ways to detect spoofed fingers. Here’s a recent example of presentation attack detection (liveness detection) from TECH5:
TECH5 participated in the 2023 LivDet Non-contact Fingerprint competition to evaluate its latest NN-based fingerprint liveness detection algorithm and has achieved first and second ranks in the “Systems” category for both single- and four-fingerprint liveness detection algorithms respectively. Both submissions achieved the lowest error rates on bonafide (live) fingerprints. TECH5 achieved 100% accuracy in detecting complex spoof types such as Ecoflex, Playdoh, wood glue, and latex with its groundbreaking Neural Network model that is only 1.5MB in size, setting a new industry benchmark for both accuracy and efficiency.
From https://tech5.ai/tech5s-mobile-fingerprint-liveness-detection-technology-ranked-the-most-accurate-in-the-market/
TECH5 excelled in detecting fake fingers for “non-contact” reading where the fingers don’t even touch a surface such as an optical surface. That’s appreciably harder than detecting fake fingers that touch contact devices.
I should note that LivDet is an independent assessment. As I’ve said before, independent technology assessments provide some guidance on the accuracy and performance of technologies.
So gummy fingers and future threats can be addressed as they arrive.
But at least gummy fingers aren’t racist.
Example 2: Gender shades
In 2017-2018, the Algorithmic Justice League set out to answer this question:
How well do IBM, Microsoft, and Face++ AI services guess the gender of a face?
From http://gendershades.org/. Yes, that’s “http,” not “https.” But I digress.
Let’s stop right there for a moment and address two items before we continue. Trust me; it’s important.
- This study evaluated only three algorithms: one from IBM, one from Microsoft, and one from Face++. It did not evaluate the hundreds of other facial recognition algorithms that existed in 2018 when the study was released.
- The study focused on gender classification and race classification. Back in those primitive innocent days of 2018, the world assumed that you could look at a person and tell whether the person was male or female, or tell the race of a person. (The phrase “self-identity” had not yet become popular, despite the Rachel Dolezal episode which happened before the Gender Shades study). Most importantly, the study did not address identification of individuals at all.
However, the findings did find something:
While the companies appear to have relatively high accuracy overall, there are notable differences in the error rates between different groups. Let’s explore.
All companies perform better on males than females with an 8.1% – 20.6% difference in error rates.
All companies perform better on lighter subjects as a whole than on darker subjects as a whole with an 11.8% – 19.2% difference in error rates.
When we analyze the results by intersectional subgroups – darker males, darker females, lighter males, lighter females – we see that all companies perform worst on darker females.
From http://gendershades.org/overview.html
What does this mean? It means that if you are using one of these three algorithms solely for the purpose of determining a person’s gender and race, some results are more accurate than others.
Three algorithms do not predict hundreds of algorithms, and classification is not identification. If you’re interested in more information on the differences between classification and identification, see Bredemarket’s November 2021 submission to the Department of Homeland Security. (Excerpt here.)
And all the stories about people such as Robert Williams being wrongfully arrested based upon faulty facial recognition results have nothing to do with Gender Shades. I’ll address this briefly (for once):
- In the United States, facial recognition identification results should only be used by the police as an investigative lead, and no one should be arrested solely on the basis of facial recognition. (The city of Detroit stated that Williams’ arrest resulted from “sloppy” detective work.)
- If you are using facial recognition for criminal investigations, your people had better have forensic face training. (Then they would know, as Detroit investigators apparently didn’t know, that the quality of surveillance footage is important.)
- If you’re going to ban computerized facial recognition (even when only used as an investigative lead, and even when only used by properly trained individuals), consider the alternative of human witness identification. Or witness misidentification. Roeling Adams, Reggie Cole, Jason Kindle, Adam Riojas, Timothy Atkins, Uriah Courtney, Jason Rivera, Vondell Lewis, Guy Miles, Luis Vargas, and Rafael Madrigal can tell you how inaccurate (and racist) human facial recognition can be. See my LinkedIn article “Don’t ban facial recognition.”
Obviously, facial recognition has been the subject of independent assessments, including continuous bias testing by the National Institute of Standards and Technology as part of its Face Recognition Vendor Test (FRVT), specifically within the 1:1 verification testing. And NIST has measured the identification bias of hundreds of algorithms, not just three.
In fact, people that were calling for facial recognition to be banned just a few years ago are now questioning the wisdom of those decisions.
But those days were quaint. Men were men, women were women, and artificial intelligence was science fiction.
The latter has certainly changed.
Example 3: Voice spoofs
Perhaps it’s an exaggeration to say that recent artificial intelligence advances will change the world. Perhaps it isn’t. Personally I’ve been concentrating on whether AI writing can adopt the correct tone of voice, but what if we take the words “tone of voice” literally? Let’s listen to President Richard Nixon:
Richard Nixon never spoke those words in public, although it’s possible that he may have rehearsed William Safire’s speech, composed in case Apollo 11 had not resulted in one giant leap for mankind. As noted in the video, Nixon’s voice and appearance were spoofed using artificial intelligence to create a “deepfake.”
It’s one thing to alter the historical record. It’s another thing altogether when a fraudster spoofs YOUR voice and takes money out of YOUR bank account. By definition, you will take that personally.
In early 2020, a branch manager of a Japanese company in Hong Kong received a call from a man whose voice he recognized—the director of his parent business. The director had good news: the company was about to make an acquisition, so he needed to authorize some transfers to the tune of $35 million. A lawyer named Martin Zelner had been hired to coordinate the procedures and the branch manager could see in his inbox emails from the director and Zelner, confirming what money needed to move where. The manager, believing everything appeared legitimate, began making the transfers.
What he didn’t know was that he’d been duped as part of an elaborate swindle, one in which fraudsters had used “deep voice” technology to clone the director’s speech…
From https://www.forbes.com/sites/thomasbrewster/2021/10/14/huge-bank-fraud-uses-deep-fake-voice-tech-to-steal-millions/?sh=8e8417775591
Now I’ll grant that this is an example of human voice verification, which can be as inaccurate as the previously referenced human witness misidentification. But are computerized systems any better, and can they detect spoofed voices?
Well, in the same way that fingerprint readers worked to overcome gummy bears, voice readers are working to overcome deepfake voices. Here’s what one company, ID R&D, is doing to combat voice spoofing:
IDVoice Verified combines ID R&D’s core voice verification biometric engine, IDVoice, with our passive voice liveness detection, IDLive Voice, to create a high-performance solution for strong authentication, fraud prevention, and anti-spoofing verification.
Anti-spoofing verification technology is a critical component in voice biometric authentication for fraud prevention services. Before determining a match, IDVoice Verified ensures that the voice presented is not a recording.
From https://www.idrnd.ai/idvoice-verified-voice-biometrics-and-anti-spoofing/
This is only the beginning of the war against voice spoofing. Other companies will pioneer new advances that will tell the real voices from the fake ones.
As for independent testing:
- ID R&D has participated in multiple ASVspoof tests, and performed well in them.
- NIST has long conducted speaker recognition evaluations. Perhaps future tests will be expanded to check for deepfakes, in the same way that the FRVT 1:1 test was expanded to check for bias.
A final thought
Yes, fraudsters can use advanced tools to do bad things.
But the people who battle fraudsters can also use advanced tools to defeat the fraudsters.
Take care of yourself, and each other.
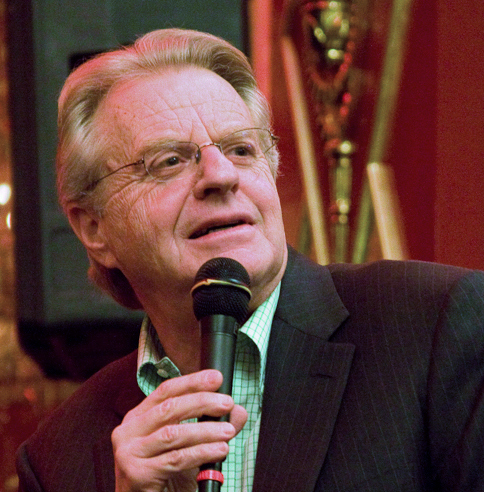
8 Comments